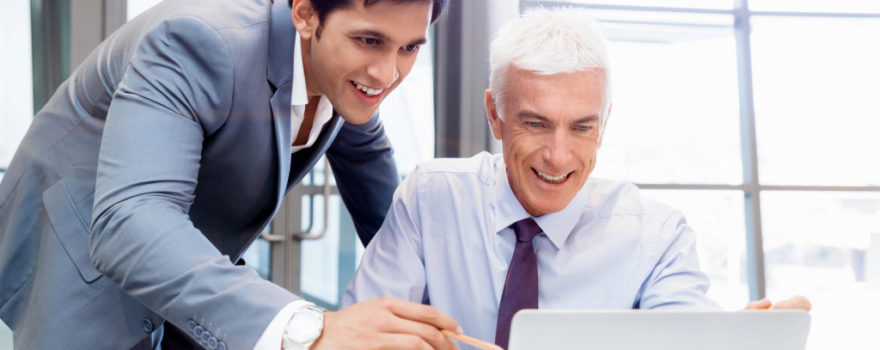
As artificial intelligence informs more decisions, companies’ AI systems must be understood by users and those affected by AI use. Actions in two areas can maximize AI’s benefits and minimize risk.
Businesses increasingly rely on artificial intelligence (AI) systems to make decisions that can significantly affect individual rights, human safety, and critical business operations. But how do these models derive their conclusions? What data do they use? And can we trust the results?
Addressing these questions is the essence of “explainability,” and getting it right is becoming essential. While many companies have begun adopting basic tools to understand how and why AI models render their insights, unlocking the full value of AI requires a comprehensive strategy. Our research finds that companies seeing the biggest bottom-line returns from AI—those that attribute at least 20 percent of EBIT to their use of AI—are more likely than others to follow best practices that enable explainability.1 Further, organizations that establish digital trust among consumers through practices such as making AI explainable are more likely to see their annual revenue and EBIT grow at rates of 10 percent or more.2
Even as explainability gains importance, it is becoming significantly harder. Modeling techniques that today power many AI applications, such as deep learning and neural networks, are inherently more difficult for humans to understand. For all the predictive insights AI can deliver, advanced machine learning engines often remain a black box. The solution isn’t simply finding better ways to convey how a system works; rather, it’s about creating tools and processes that can help even the deep expert understand the outcome and then explain it to others.
To shed light on these systems and meet the needs of customers, employees, and regulators, organizations need to master the fundamentals of explainability. Gaining that mastery requires establishing a governance framework, putting in place the right practices, and investing in the right set of tools.
What makes explainability challenging
Explainability is the capacity to express why an AI system reached a particular decision, recommendation, or prediction. Developing this capability requires understanding how the AI model operates and the types of data used to train it. That sounds simple enough, but the more sophisticated an AI system becomes, the harder it is to pinpoint exactly how it derived a particular insight. AI engines get “smarter” over time by continually ingesting data, gauging the predictive power of different algorithmic combinations, and updating the resulting model. They do all this at blazing speeds, sometimes delivering outputs within fractions of a second.
Disentangling a first-order insight and explaining how the AI went from A to B might be relatively easy. But as AI engines interpolate and reinterpolate data, the insight audit trail becomes harder to follow.
Complicating matters, different consumers of the AI system’s data have different explainability needs. A bank that uses an AI engine to support credit decisions will need to provide consumers who are denied a loan with a reason for that outcome. Loan officers and AI practitioners might need even more granular information to help them understand the risk factors and weightings used in rendering the decision to ensure the model is tuned optimally. And the risk function or diversity office may need to confirm that the data used in the AI engine are not biased against certain applicants. Regulators and other stakeholders also will have specific needs and interests.
Five ways explainable AI can benefit organizations
Mastering explainability helps technology, business, and risk professionals in at least five ways
- Increasing productivity. Techniques that enable explainability can more quickly reveal errors or areas for improvement, making it easier for machine learning operations (MLOps) teams tasked with supervising AI systems to monitor and maintain AI systems efficiently. As an example, understanding the specific features that lead to the model output helps technical teams confirm whether patterns identified by the model are broadly applicable and relevant to future predictions or instead reflect one-off or anomalous historical data.
- Building trust and adoption. Explainability is also crucial to building trust. Customers, regulators, and the public at large all need to feel confident that the AI models rendering consequential decisions are doing so in an accurate and fair way. Likewise, even the most cutting-edge AI systems will gather dust if intended users don’t understand the basis for the recommendations being supplied. Sales teams, for instance, are more apt to trust their gut over an AI application whose suggested next-best actions seem to come from a black box. Knowing why an AI application made its recommendation increases sales professionals’ confidence in following it.
- Surfacing new, value-generating interventions. Unpacking how a model works can also help companies surface business interventions that would otherwise remain hidden. In some cases, the deeper understanding into the why of a prediction can lead to even more value than the prediction or recommendation itself. For example, a prediction of customer churn in a certain segment can be helpful by itself, but an explanation of why the churn is likely can reveal the most effective ways for the business to intervene. For one auto insurer, using explainability tools such as SHAP values revealed how greater risk was associated with certain interactions between vehicle and driver attributes. The company used these insights to adjust its risk models, after which its performance improved significantly.
- Ensuring AI provides business value. When the technical team can explain how an AI system functions, the business team can confirm that the intended business objective is being met and spot situations where something was lost in translation. This ensures that an AI application is set up to deliver its expected value.
- Mitigating regulatory and other risks. Explainability helps organizations mitigate risks. AI systems that run afoul of ethical norms, even if inadvertently, can ignite intense public, media, and regulatory scrutiny. Legal and risk teams can use the explanation provided by the technical team, along with the intended business use case, to confirm the system complies with applicable laws and regulations and is aligned with internal company policies and values.
In some sectors, explainability is a requirement. For example, a recent bulletin issued by the California Department of Insurance requires insurers to explain adverse actions taken based on complex algorithms.3 As use of AI grows, organizations can expect more rules concerning explainability. New regulations, such as the draft EU AI regulation, may contain specific explainability compliance steps. Even when not specifically mandated, companies will need to confirm that any tool used to render actions such as credit determinations comply with applicable antidiscrimination laws, as well as laws prohibiting unfair or deceptive practices.
How businesses can make AI explainable
Organizations that build a framework for explainability and acquire the right enabling tools will be better positioned to capture the full value of deep learning and other AI advances. We suggest organizations start by including explainability as one of the key principles within their responsible AI guidelines. Then organizations can operationalize this principle by establishing an AI governance committee to set standards and guidance for AI development teams, including guidelines for use-case-specific review processes, and by investing in the right talent, technology, research, and training.
Establish an AI governance committee to guide AI development teams
Establishment of an AI governance committee includes recruiting its members and defining the scope of work. The explainability and risk assessment of AI use cases may be complex, requiring an understanding of the business objective, the intended users, the technology, and any applicable legal requirements. For this reason, organizations will want to convene a cross-functional set of experienced professionals, including business leaders, technical experts, and legal and risk professionals. Bringing in diverse points of view internally and externally can also help the company test whether the explanations developed to support an AI model are intuitive and effective for different audiences.
A key function of the committee will be setting standards for AI explainability. As part of the standards-setting process, effective AI governance committees often establish a risk taxonomy that can be used to classify the sensitivity of different AI use cases. The taxonomy links to guidance that outlines expectations and standards with respect to different use cases. For example, is an explanation necessary to comply with regulatory requirements, or is the goal simply to provide an overview of functionality to aid adoption? The taxonomy also clarifies when escalation to a review board or legal may be required.
Because each AI use case can present a different set of risks and legal requirements related to explainability, organizations should establish a process for model development teams to assess each use case. This process better positions the organization to manage these risks and capture value from AI. Tracking the outcome of these assessments within a central inventory helps ensure the organization can monitor the use of AI systems for compliance with law and adherence to responsible AI principles.
As part of the review process, teams will need to consider whether to go beyond the basic explainability requirements, based on the potential value resulting from, for example, greater trust, adoption, or productivity. In some cases, a trade-off may exist between explainability and accuracy. For example, simplifying an AI model’s mechanics might improve user trust, but in some—not all—cases, a shift might make the model less accurate. When trade-offs exist, teams will need to weigh the competing considerations, including any regulatory requirements, and escalate to leadership as necessary.
Teams may be able to address these trade-offs themselves. Sometimes they can reverse-engineer the factors driving predictive outcomes for advanced AI models by tracking model performance and discerning patterns. They can then try to replicate the complex model using simpler and better-understood statistical methods such as logistic regression. In some cases, the result will be an equally high-performing model with outputs that are inherently explainable.
Invest in the right talent, explainability technology, research, and training
The rapid pace of technological and legal change within the area of explainability makes it urgent for companies to hire the right talent, invest in the right set of tools, engage in active research, and conduct ongoing training.
High-performing organizations develop a talent strategy to support AI governance across the enterprise. These companies seek to retain legal and risk colleagues who can actively and meaningfully engage with both the business and technologists to navigate applicable regulations, meet consumer expectations, and “future-proof” core products (including features and data sets) as the law evolves. Similarly, companies are well served to hire technologists familiar with legal issues or focused on technology ethics.
Investment in explainability technology should aim to acquire appropriate tools for meeting the needs identified by development teams during the review process. For example, more advanced tooling may provide a robust explanation in a context that would otherwise require teams to sacrifice accuracy. Although the up-front cost of bespoke solutions may be higher, it sometimes pays off in the long run because they can take into account the context in which the model is being deployed, including the intended users and any legal or regulatory requirements. Companies considering off-the-shelf and open-source tools should understand any limitations of these options. For example, some explainability tools rely on post-hoc explanations that deduce the relevant factors based only on a review of the system output. If this limited approach yields a less-than-accurate explanation of the causal factors driving the outcome, users’ confidence in the system output might be unwarranted.
Research is an ongoing requirement because legal and regulatory requirements, as well as consumer expectations and industry norms, are changing rapidly. AI governance committees will want to actively monitor and, where possible, conduct their own research in this space to ensure continual learning and knowledge development. The committee should also establish a training program to ensure employees across the organization understand and are able to apply the latest developments in this space.